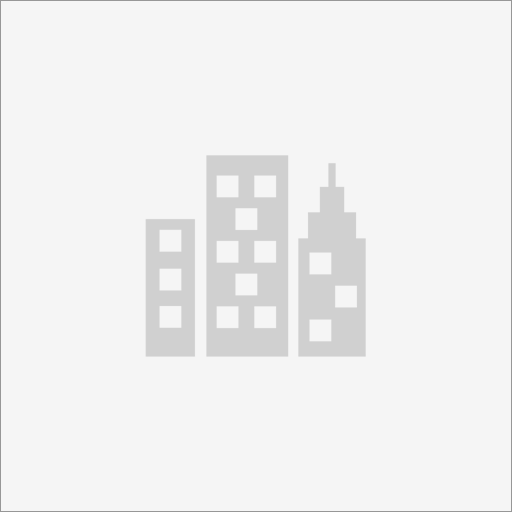
University of Sunderland
About the Project
Fully funded PhD studentship in applied Machine Learning [UK and international]
Applications are invited for a fully funded studentship to start in October 2024
Project Overview: This project aims to develop Machine Learning (ML) models that accurately model the performance of the healthcare providers. These models would serve as invaluable tools for optimizing operational processes and facilitating the delivery of patient-centred care. Subsequently, these models will be utilized to investigate the impact of fatigue on the effectiveness of healthcare providers, with a particular emphasis on the influence of daylight-saving time (DST) transitions. While some evidence exists regarding the detrimental effects of DST on health outcomes, its specific implications for healthcare providers’ effectiveness remain uncertain. Through rigorous data analysis and the integration of ML algorithms, this study aims to bridge this knowledge gap, uncovering the potential links between DST transitions, fatigue, and healthcare providers’ performance. By clarifying these relationships, the findings will not only contribute to the existing literature but also offer critical insights for healthcare organizations and professionals in designing interventions and strategies to mitigate the adverse effects of DST transitions, ultimately enhancing the quality and safety of patient care. This project is done in collaboration between Dr Basel Barakat, an expert in ML and the primary supervisor for this project, and Dr Aiste Steponenaite, a Circadian Neuroscientist from University of Kent who will act as an external supervisor.
In addition to completing the PhD, this studentship will also entail up to 6 hours per week of supporting teaching demonstrations in workshops. This commitment is designed to offset the fees and offer an opportunity to gain valuable teaching experience. It aims to foster an environment of support and active engagement within student workshops, allowing for first-hand experience and skill development in teaching.
Eligibility Criteria: The candidate should have at least first class or upper second-class honours degree in Computer Science or related discipline, with demonstrable machine learning skills. The candidate should have a passion for machine learning models development and be able to complement their software development skills (Python preferable) with a strong focus on time series forecasting. The project would involve dealing with large amounts of unstructured data, thus having some experience in such projects would be desirable.
Funding: This PhD studentship is fully funded at the Home Student rate. International students can apply, but you will need to pay the difference between Home and International fees (currently £9000 per year). A successful candidate will also receive a UKRI rate stipend for living expenses (the stipend for the academic year 2023-2024 is currently set at £18,622). Students who have a Settled Status will be considered as Home Students.
Requirements
- BSc in Computer science or related program – Essential
- MSc in Machine learning or related program – Desirable
- Excellent programming and problem-solving skills – Essential
- Demonstrable experience in Machine Learning – Essential
- Skills in time series forecasting – Desirable
- Good communication skills – Essential
- Appropriate IELTS score, if required – Essential
Academic queries:
If you have any questions, please contact project supervisor Dr Basel Barakat via email [email protected]
If you are interested please send your CV and a cover letter to Dr Basel Barakat.
To help us track our recruitment effort, please indicate in your email – cover/motivation letter where (globalvacancies.org) you saw this job posting.