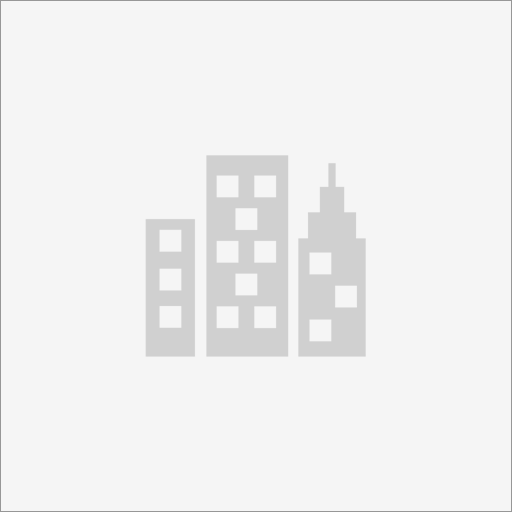
University of Leicester
About the Project
GTA funded PhD studentship in Computing
Highlights
- Novel vision-language models for understanding social-economic data for clinical decision-making and generating pseudo-labels for medical image classification
- Novel end-to-end approaches in translating multiple sign languages to speech for hearing-impaired individuals
- High potential for industrial impact research and international collaboration with Duke-NUS Medical school
Project
This project aims to investigate two separate but related problems for improving the overall health-related experience.
In the first problem, we explore the use of underutilised data such as the social-economic data, the unlabelled medical data and the doctors’ diagnosis (in textual form) for clinical decision-making and medical image classification.
Existing studies reported that the social-economic data, e.g., the social determinants of health, has a strong association with differential health outcomes such as the effects of social support on breast cancer risk and survival. This information is commonly found in electronic clinical notes, either on electronic documents, or in electronic health records (EHR) databases. Unfortunately, it is unstructured due to a lack of standardized data elements, and different data collection practices, which limit its access. Recent studies attempted to use NLP technologies to unlock and analyse this information, but the type of social-economic data studied is limited and inconsistent.
On the other hand, the development of fully automatic medical image classification requires massive amounts of high quality annotated medical images, which are prohibitively expensive and time-consuming. We found correlations between the electronic clinical notes (the same set of electronic clinical notes used above for extracting and understanding the social-economic data) and the medical imaging data, and we aim to further exploit it through generating pseudo-labels for medical image classification.
We aim to create novel NLP models that can understand, extract, and analyse a comprehensive social-economic data and other important textual information found in electronic documents and EHR databases for clinical decision-making and medical image classification. We will create several novel NLP models including models to represent the social and medical information and models to generate pseudo-labels from electronic clinical notes.
In the second problem, we explore the use of translating signed languages to text/speech. Existing studies mainly focus on American Signed Language. However, there are over 200 different signed languages and up to 70 million deaf people. The communication between a normal and a deaf individual also proved challenging, largely due to the lack of knowledge to understand/hear each other language. Note: the first problem work is also applicable for hearing-impaired individuals.
We aim to create a novel multilingual signed languages model that can perform text/speech translation.
The research technology output can be transferred to real-world environment such as Leicester Biomedical Research Centre.
The student can potentially work in a collaboration environment with the supervisor’s collaborator from Duke-NUS Medical school.
PhD start date 23 September 2024
Enquiries to project supervisor Dr Fuxiang Chen [email protected] or [email protected]
Further details and application advice at https://le.ac.uk/study/research-degrees/funded-opportunities/cms-gta
To help us track our recruitment effort, please indicate in your email – cover/motivation letter where (globalvacancies.org) you saw this job posting.