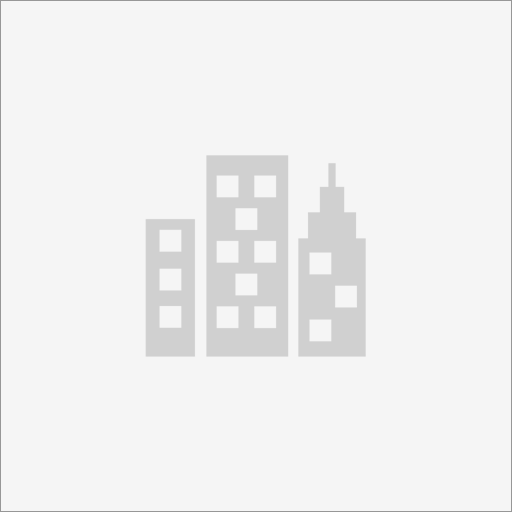
University of Cambridge
About the Project
The UKRI AI Centre for Doctoral Training (CDT) in Decision Making for Complex Systems is a joint CDT between The University of Manchester and the University of Cambridge. The CDT provides funding for four years of advanced studies towards a PhD. The first year consists of a taught program at Manchester that will cover the fundamentals of Machine Learning. This year is followed by three years of research at either at Manchester or Cambridge. Please note the research element of the PhD will take place at the host institution of the supervisor listed for each project.
Neural emulation has risen to prominence in the last decade as a tool for AI-accelerating computationally expensive scientific models. Tools such as PICO [astro-ph/0606709], SkyNet [1309.0790], CosmoPower [2106.03846], and globalemu [2104.04336] have a successful history of enabling cosmological investigation by turning costly analyses requiring supercomputers to ones that can be done on a laptop. Beyond cosmology, emulators become all the more relevant for making real-time sub-second decisions in a principled Bayesian manner.
This PhD project will build on recent work that has shown nested sampling [2205.15570] offers unique advantages in the training of emulators [2312.00294]. The strategy by which nested sampling scans high-dimensional parameter spaces (starting from the deep tails of an objective function and focusing inward in a self-similar compression) is ideally suited for providing targeted training data for emulators, rendering them capable of emulating across far wider dynamic ranges than traditional approaches (e.g. as applied to HERA simulations [2108.07282]). Initial projects will begin developing on work begun with the BAMBI algorithm [1110.2997], for modern cosmological datasets, supported by the group’s DiRAC allocations. The direct results of such projects will then be applied to Bayesian model comparison to both cosmological theories and Bayesian experimental design (e.g. REACH [2210.07409]), in which Bayes theorem acts as the ultimate arbiter in decision-making.
Projects building from this will then apply these emulation techniques to real-time problems beyond cosmology, in particular to the group’s ongoing research in adversarial machine learning and nested active learning. There will also be opportunities to apply these emulators in the group’s ongoing ERC research programme in simulation based inference [ERC]. Other topics available involve using nested sampling itself to train compromise-free Bayesian neural networks [2004.12211] [2205.11151], with the potential to provide emulation.
The Cambridge astrophysics group is a centre of expertise in nested sampling, machine learning and cosmology. In addition, there is opportunity to work with the group’s spin-out company PolyChord Ltd in applying these tools to industry problems, for example in Electric vehicle battery design, with existing collaborations in the group between AI-driven materials science and with the GAMBIT particle physics collaboration [GAMBIT].
Entry requirements
Applicants should have, or expect to achieve, at least a 2.1 honours degree or a master’s (or international equivalent) in a relevant science or engineering related discipline.
How to Apply
As the CDT has only recently been awarded we strongly encourage you to contact the supervisor of the project you are interested in with your CV and supporting documents. You will have a chance to meet with prospective supervisors prior to submitting an application – further details will be provided.
Equality, diversity and inclusion is fundamental to the success of The University of Manchester, and is at the heart of all of our activities. We know that diversity strengthens our research community, leading to enhanced research creativity, productivity and quality, and societal and economic impact.
We actively encourage applicants from diverse career paths and backgrounds and from all sections of the community, regardless of age, disability, ethnicity, gender, gender expression, sexual orientation and transgender status.
We also support applications from those returning from a career break or other roles. We consider offering flexible study arrangements (including part-time: 50%, 60% or 80%, depending on the project/funder).
AI_CDT_DecisionMaking
To help us track our recruitment effort, please indicate in your email – cover/motivation letter where (globalvacancies.org) you saw this job posting.