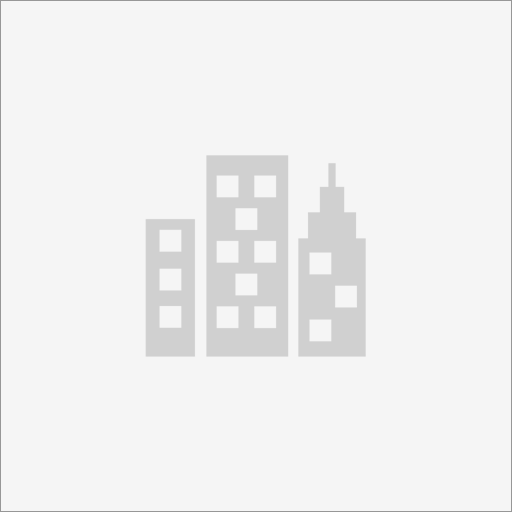
University of Leicester
About the Project
Project Highlights
- Integration of medical data streams
- Physics-informed neural networks
- Clinical decision support systems
Project
Developing accurate digital twins requires the skilful combination of mathematical modelling, numerical simulations, software development, and application insight. In this project, we will build on the recently developed capability to seamlessly fuse finite element solvers and neural network architectures [1] to build truthful and versatile digital twins for Lung Disease Management. Key to this will be leveraging automated differentiation and state-of-the-art PDE-constrained optimization methods [2] to allow rapid updating-cycles of the digital twin as new data becomes available after deployment.
This highly-interdisciplinary project will help develop AI-based digital twins (DTs) of patients’ lungs to predict disease progression and optimize treatment strategies. A DT is a distributed computational model of the system to be twinned (in our case the lung of a human patient) that is connected to the system in a bidirectional fashion, i.e., periodically recalibrated with patient data and providing patient predictions and treatment suggestions over time.
This approach requires three main modular components that are continuously updated over time: (1) data acquisition (for example imaging, clinical history, demographics, genomics data, and data from low-cost wearable devices); (2) modelling and simulation (based on anatomy and physiology and fitted to the patient characteristics); and (3) clinical decision making.
The GTA can choose one or more aspects to focus on according to their interests and inclinations.
The GTA will also gain insights into and familiarise with the lung physiopathology models [3] and the health data collected from imaging, physiological measurements, and diagnostic tests. AI techniques, including physics-informed neural networks [4] and convolutional neural networks, will be employed to process, harmonise, and analyse medical data. Using data assimilation and diffusion based models that integrate physics-based insights from biomechanics and physiology based computational approach of lung as well as the machine learning process, the candidate will help in developing predictive models to generate physically realistic predictions. This is expected to enhance the accuracy and efficiency of lung disease diagnosis and inform clinical decision support systems to improve patients’ health.
This interdisciplinary research project is jointly supervised by academics with expertise in distributed machine learning, applied mathematics, finite element modelling, PDE-constrained optimization, and medical sciences. The PhD student will also have the opportunity to collaborate closely with established industrial partners to apply their knowledge to real-world applications
Project enquiries to supervisor: Prof Ashiq Anjum a.anjum@leicester.ac.uk
General enquiries CMSpgr@le.ac.uk
How to Apply
Please refer to the application advice and use the application link on our web page
https://le.ac.uk/study/research-degrees/funded-opportunities/cms-gta
To help us track our recruitment effort, please indicate in your email – cover/motivation letter where (globalvacancies.org) you saw this job posting.